Multimodal Generative AI: a solution to drug authenticity concerns
Multimodal Generative AI: a solution to drug authenticity concerns
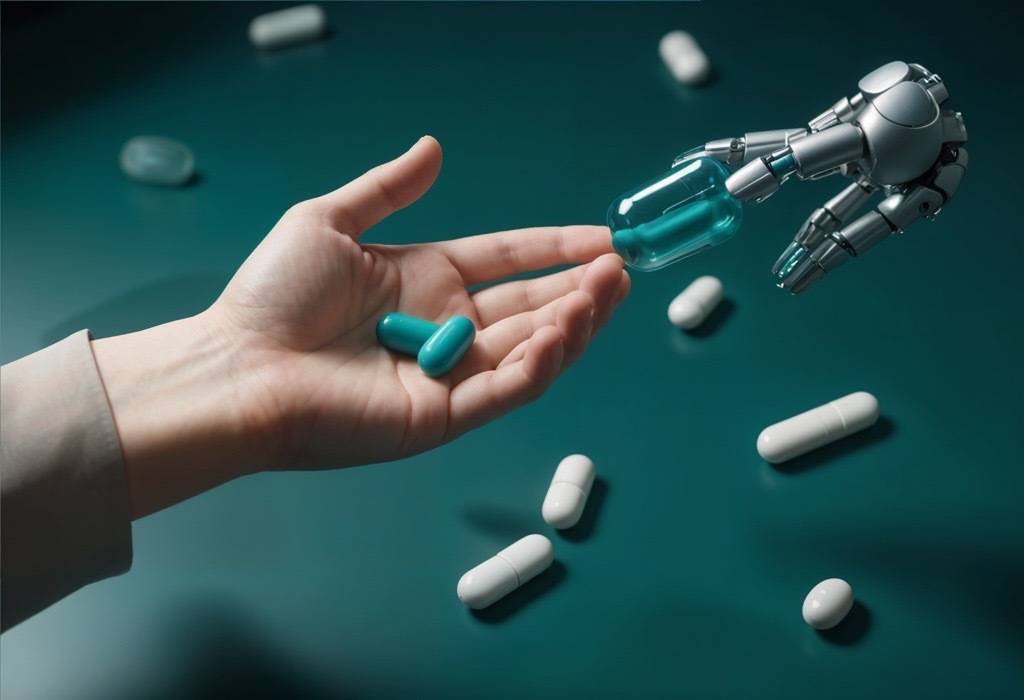
At a time when public health is under constant threat from drug counterfeiting and the imitation of dangerous molecules, technological innovation is emerging as a life-saving solution. Recent advances in generative and multimodal artificial intelligence (AI) offer promising solutions for strengthening the authenticity of medicines and protecting the image of pharmaceutical groups. However, these technologies also raise critical questions about their implementation and regulation.
Emerging Trends in Generative and Multimodal AI
Generative AI, enabling the creation of new and realistic content from existing data, and multimodal AI, capable of understanding and generating information from multiple data sources, are redefining the pharmaceutical landscape. These revolutionary technologies can analyze complex, often massive, and heterogeneous datasets with unprecedented accuracy and speed, producing accurate models to identify genuine medicines and detect counterfeits.
Generative AI uses advanced neural networks to simulate the chemical and physical properties of drugs, creating digital twins that can be compared with suspect samples to detect subtle anomalies. In addition, multimodal AI integrates and analyses data from a variety of sources, such as manufacturing reports, pharmacovigilance databases, spectroscopic analysis, and IoT sensors, to provide a holistic and integrated view of each drug batch. This ability to interconnect and contextualize disparate information not only helps to verify the authenticity of medicines but also to detect anomalies in the supply chain and prevent the distribution of counterfeit substances. By combining the power of new data generation and multimodal analysis, these technologies offer a robust defense against the growing threat of counterfeit drugs, while ensuring regulatory compliance and patient safety.
Generative AI applications
Advances in generative and multimodal AI are paving the way for a multitude of applications in the pharmaceutical industry. Here are some of the most promising ways in which these technologies can transform drug safety and traceability.
- Drug Authenticity Validation: Generative AI can simulate the chemical and physical properties of authentic medicines, enabling a fine comparison with suspect samples. Algorithms can detect minute discrepancies that are often missed by traditional methods. In addition, spectroscopic analysis tools integrated with AI models enable real-time verification, improving the speed and efficiency of inspections.
- Traceability and Transparency: The integration of multimodal AI into pharmaceutical supply chains makes it possible to track every stage in the production and distribution of medicines. IoT sensors and blockchain databases, combined with AI analysis, provide foolproof traceability. This approach ensures that every batch of medicine can be traced back to its source, reducing the risk of falsification and increasing the confidence of consumers and regulators.
- Molecule Emulation Identification: The ability of generative AI to model molecules enables the prediction of dangerous variations and the rapid identification of non-compliant emulations. This proactive prevention is crucial to avoid the distribution of potentially harmful substances. AI models can also anticipate possible mutations in molecules and propose solutions to neutralize their harmful effects before they reach the market.
- Predictive and preventive analysis: Multimodal AI makes it possible to combine clinical data, pharmacovigilance reports, and epidemiological information to predict geographical areas or populations at risk of exposure to counterfeit medicines. This approach makes it possible to target interventions and allocate resources more effectively for risk prevention.
Implications and Challenges
Despite their revolutionary power, generative and multimodal AI technologies pose significant challenges that cannot be ignored. The accuracy of algorithms is linked to the quality of the data used to train them. Biased or insufficient data can lead to costly and potentially dangerous errors. For example, an AI model trained on unrepresentative samples could fail to detect certain sophisticated counterfeits, thereby compromising patient safety. This data quality issue is exacerbated by the variability of data collection practices worldwide, making it difficult to harmonize quality standards.
In addition, the complexity of generative models raises questions about the transparency and understanding of AI decision-making processes. Healthcare professionals and regulators not only need to understand how these algorithms arrive at their conclusions, but they also need to be able to verify and validate these decisions to guarantee their reliability and compliance with ethical and legal standards.
The regulation of these new technologies is also complex and difficult. Public health authorities need to develop and implement robust frameworks to oversee the use of AI in the pharmaceutical industry. This includes establishing rigorous validation protocols for AI algorithms, regular system audits to ensure ongoing performance, and clear data use and sharing guidelines. These regulations need to be flexible enough to adapt to rapid changes in technology, yet strict enough to ensure safety and ethics.
Read more : Navigating generative AI in drug discovery and data analysis: Seizing the opportunity and avoiding pitfalls
Teamwork and Training
The successful integration of generative and multimodal AI in the battle against counterfeit medicines also relies on close collaboration between the various players in the sector. Pharmaceutical groups, researchers, regulators and healthcare professionals must work together to share best practices, data, and tools.
In addition, ongoing training for healthcare professionals and transparent communication with the public are essential to build trust in these innovative technologies. Doctors, pharmacists, and other players in the distribution chain must be trained in the use of AI tools and understand their limits and potential.
The introduction of generative and multimodal AI represents a major advance for public health and drug safety. This technology offers powerful solutions to combat the challenges of drug authenticity. However, it is crucial to approach these advances with a critical and cautious approach, putting in place adequate safeguards to protect public health and the integrity of the pharmaceutical industry. The road to widespread, secure use of these technologies is fraught with pitfalls, but the potential benefits for public health and consumer confidence amply justify the effort and investment required.