DeepSeek V3 + Jetson Orin Nano: A revolution for embedded AI in pharma?
PIXELS LAB has tested for you the combination of two major innovations: DeepSeek V3 and Jetson Orin Nano
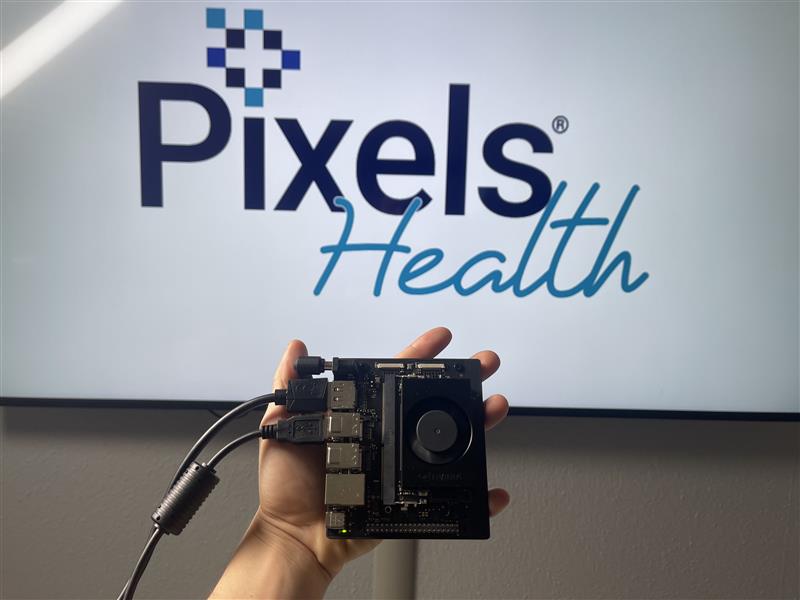
PIXELS LAB has tested for you the aggregation of two major innovations: DeepSeek V3 and Jetson Orin Nano – Discover our analysis and conclusions!
At Pixels Lab, our mission is to explore the most advanced innovations and identify how they can transform demanding sectors like healthcare and pharma.
Today, we’ve tested an unprecedented combination for you:
DeepSeek V3, one of the most advanced open-source AI models on the market. Thanks to its advanced architecture and training on massive datasets, it stands out for its versatility, accuracy and ability to adapt to the specific needs of companies and demanding industries such as healthcare and pharma.
Jetson Orin Nano, is one of NVIDIA’s most powerful and energy-efficient AI gas pedals, offering impressive computing power in a compact, energy-optimized form factor.
Why this test? Because this aggregation of two disruptive technologies could drastically reduce AI access costs, improve laboratory automation, and accelerate pharmaceutical innovation and clinical research.
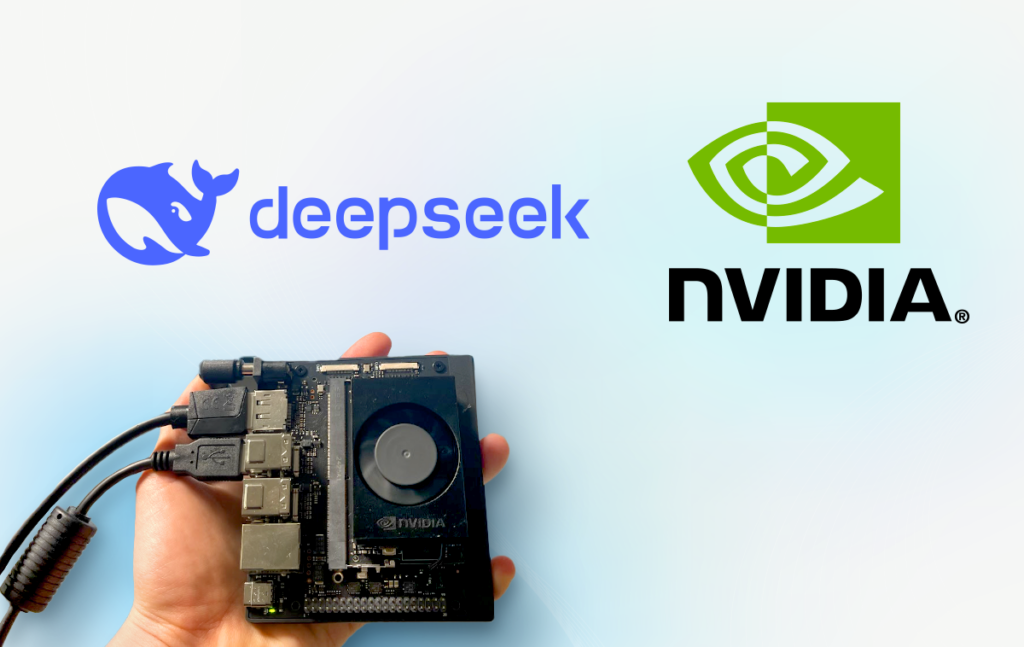
DeepSeek V3 vs ChatGPT vs Gemini: Performance comparison
20x lower training cost than competing models:
DeepSeek V3 redefines the economics of artificial intelligence by optimizing training costs. Thanks to advanced algorithms and an efficient architecture, this model requires 20 times fewer resources than competing solutions to achieve equivalent or even superior performance. This drastic reduction in costs gives companies access to powerful AI without massive infrastructure investment, making cutting-edge models more accessible to a wider range of players.
Increased scalability, with 500 simultaneous queries:
One of DeepSeek V3’s major assets is its ability to handle 500 simultaneous queries, guaranteeing a fast, fluid response even under heavy load. Unlike traditional models, which show limitations when scaling up, DeepSeek V3 is designed for massive real-time execution, making it ideal for applications requiring high availability, such as virtual assistants, data analysis or intensive cloud services.
Lower power consumption, ideal for embedded integration:
DeepSeek V3 also stands out for its low energy consumption, a key factor for its adoption in constrained environments such as edge computing or embedded systems. Its optimized architecture reduces GPU and CPU consumption, enabling efficient integration on low-power devices such as smartphones, connected objects and medical equipment. This opens up new prospects for embedded AI solutions, combining high performance and energy efficiency.
This test proves that it is possible to access high-performance AI without a costly infrastructure.
We measured the efficiency of DeepSeek V3 against GPT-4 (ChatGPT) and Gemini, evaluating their performance on a Jetson Orin Nano with JetPack 6.
But beyond technological performance, there remains a major challenge: Adoption.
Why is AI still struggling to establish itself in pharma?
While artificial intelligence platforms are multiplying, user engagement remains a major challenge. 70% of users are not engaged with these solutions. Why is this?
5 major obstacles to the adoption of AI in pharma :
1 .Lack of habit and training
Many healthcare professionals and researchers are not used to using AI on a daily basis. The learning curve is still too high.
2. Strict and constantly evolving regulations
The regulatory framework in pharma (FDA, EMA, ANSM) is a major brake. AI cannot be integrated without strict validation, which slows down its adoption.
3. Implementation costs still high
Even if training costs are coming down, implementing an AI model in a secure environment, validated and integrated with existing processes remains a substantial investment.
4. A need for transparency and interpretability
In the pharmaceutical field, every decision must be traceable and justifiable. Yet AI is still perceived as a “black box” that is difficult to explain.
5. A reluctance to change and automated tools
Humans remain at the heart of medical and scientific decisions. Many experts still consider that AI cannot replace their analysis and expertise.
So the challenge is not just technological or economic, but also human, organizational and regulatory.
Embedded AI use cases for the pharmaceutical industry
Embedded AI opens up unprecedented perspectives in healthcare and research. Here are the concrete applications we have identified:
1. Accelerated clinical research and medical studies :
Automatic generation and analysis of complex scientific reports.
2. Optimized drug development :
Model molecular interactions and reduce preclinical trial times
3. Automate regulatory reporting :
Accelerate FDA and EMA compliance processes.
4. Omnichannel & HCP engagement (Healthcare professionals) :
Ultra-targeted content for medical teams and Key Opinion Leaders (KOLs).
5. AI integration in robotics and automation :
Advanced automation in research labs and biotechs.
But for AI to be truly adopted, there needs to be a change in mindset and strategic support for teams in the field.
Conclusion: a technological and economic breakthrough for pharma
Our test shows that the DeepSeek V3 + NVIDIA Jetson Orin Nano combination offers a high-performance alternative to conventional AI models.
Benefits of DeepSeek V3 + Jetson Orin Nano: High-performance, low-cost embedded AI
Ultra-powerful embedded AI:
The combination of DeepSeek V3 and the NVIDIA Jetson Orin Nano enables the deployment of autonomous artificial intelligence, capable of executing complex tasks with impressive speed.
- Advanced computing performance: : With its AI-optimized architecture, the Jetson Orin Nano leverages the capabilities of NVIDIA Ampere GPUs to accelerate DeepSeek V3 model processing.
- Drastically reduced latency: Unlike cloud models, which require data to be sent back and forth to remote servers, here processing is carried out locally, considerably improving the responsiveness and reliability of predictions
- An ideal solution for edge computing and IoT: This technological duo paves the way for embedded applications in critical fields such as healthcare, robotics, automotive, surveillance and Industry 4.0.
Costs cut by a factor of 20:
L’un des freins majeurs à l’adoption de l’IA est le coût des infrastructures nécessaires pour entraîner et exécuter des modèles avancés. L’association DeepSeek V3 + NVIDIA Jetson Orin Nano divise ces coûts par 20, offrant un retour sur investissement exceptionnel.
- Eliminate cloud costs:Hosting and running AI models in the cloud represents a significant recurring cost. With this embedded alternative, companies are freed from subscription and storage costs.
- Less energy consumption:Data centers consume a lot of energy. By running calculations locally, this solution significantly reduces carbon footprint and energy costs.
- Cost-effective, affordable hardware:The Jetson Orin Nano offers an unbeatable cost/performance ratio compared with more expensive GPU solutions or managed AI services offered by hyperscalers.
Easy access to advanced models:
Another major advantage of this solution is its ease of implementation. Unlike cloud solutions, which often require expertise in infrastructure, DevOps and data pipeline management, the DeepSeek V3 + Jetson Orin Nano combination enables rapid, accessible deployment.
- Plug-and-play configuration: thanks to an ecosystem optimized for developers, installing and running AI models becomes simple and intuitive.
- Compatibility with standard frameworks: This solution supports the most popular AI frameworks, including TensorFlow, PyTorch, ONNX and JAX, enabling easy integration into existing projects.
- Scalability to suit your needs: Whether for a pilot project or large-scale deployment, this solution adapts to a variety of use cases, enabling a gradual ramp-up.
Testing the Limits of DeepSeek on Jetson Orin Nano – A Necessary Challenge
With the rise of embedded artificial intelligence technologies, we decided to subject the DeepSeek V3 model to an ambitious test: running it on an NVIDIA Jetson Orin Nano board, known for its energy efficiency and versatility in constrained environments. With its 67 TOPS, the Jetson Orin Nano is optimized for lightweight AI applications (computer vision, real-time processing) but remains far below the capabilities of a chip like the NVIDIA H800, which is specifically designed for intensive AI workloads in data centers.
Comparatif technique: Jetson Orin Nano vs NVIDIA H800
Caractéristique | Jetson Orin Nano | NVIDIA H800 |
Puissance IA | Jusqu’à 67 TOPS | Jusqu’à 700 TFLOPS (spécialisés) |
Consommation énergétique | Faible (7W à 25W) | Élevée (plusieurs centaines de watts) |
Optimisation | Applications embarquées | Applications IA intensives |
Type d’utilisation | Vision par ordinateur, IA légère | Modèles IA de grande taille |
Prix | Environ 289 CHF (~300 $) | À partir de 30 000 $ |
Technical Comparison: Jetson Orin Nano vs. NVIDIA H800A Necessary Adaptation: DeepSeek R1 1.5B
To carry out this experiment, we opted for a lighter version of the model, DeepSeek R1 1.5B, designed for less powerful environments. This version enabled us to generate usable results while adhering to the hardware constraints of the Jetson board. The results are encouraging, particularly in scenarios involving content optimization for marketing and scientific purposes.
DeepSeek: Incremental or Disruptive Innovation?
Even in its lightweight version, DeepSeek presents interesting prospects for embedded AI. However, this raises several questions:
A Disruptive Innovation?
DeepSeek could revolutionize AI accessibility in sectors where computing power is limited. By making it possible to adapt complex models to energy-efficient platforms, it pushes the boundaries of what is achievable on embedded devices.
An Incremental Improvement?
Conversely, one could view this advancement as a gradual evolution of existing technologies. While model reduction optimization allows for some adaptation, the need for more powerful hardware remains dominant for intensive use cases.
Conclusion
In summary, if the goal is to run the full DeepSeek model with performance comparable to the H800, more powerful hardware solutions must be considered, implying a significantly higher budget. However, for applications with limited budgets, using distilled versions of the model on platforms like the Jetson Nano can be a viable alternative, albeit with some performance trade-offs.
Technology is not enough: the 2 challenges of AI adoption in pharma:
AI offers considerable opportunities to accelerate research, optimize clinical trials and improve patient care. Yet its adoption remains a major challenge. Two main obstacles are holding back its integration:
1️⃣ Adoption by professionals
2️⃣ Investment in user training and support.
For AI to become an effective transformation lever, companies need to go beyond mere technological innovation and work on these two strategic axes.
Challenge 1: Adoption by professionals
Even with major advances like DeepSeek V3 + NVIDIA Jetson Orin Nano, AI can only be effective if it is accepted and adopted by those who will use it on a daily basis. However, several obstacles remain:
- Lack of trust in AI decisions: Healthcare professionals and pharma experts want to understand how AI arrives at its results before integrating it into their decision-making process.
- Strong regulation and legal responsibility: In a sector as regulated as pharma, every innovation must guarantee compliance with strict standards (FDA, EMA, Swissmedic…). AI must be traceable and explainable to avoid any legal risk.
- Entrenched practices and resistance to change: Many experts work with tried and tested methods and have not yet integrated AI as an everyday tool. Adoption cannot be forced; it must be gradual and accompanied.
- Lack of concrete, validated examples: While AI has proven its effectiveness in certain applications, large-scale clinical studies and validation are still needed to convince professionals of its real contribution.
- The solution? Raise awareness and involve users right from the development stage of AI tools, by highlighting concrete cases and guaranteeing transparency and explicability.
Challenge 2: Invest as much in technological innovation as in user training and support.
Technological development alone is not enough. To successfully integrate AI into pharma, companies must also invest in user training and support.
- Training teams in the basics of AI: Many professionals do not yet have the knowledge they need to use these tools effectively. Appropriate training is essential.
- Create intuitive, ergonomic AI interfaces: AI must integrate naturally into existing workflows to avoid any additional complexity.
- Accompany change with experts: Accompanying programs and AI referents in companies can help allay fears and facilitate gradual adoption.
- Enhance transparency and explicability of models: To guarantee rapid adoption, AI tools need to offer clear visibility of how they work, and enable human control.