Large Behavioral Model (LBM): A Revolutionary Opportunity for the Pharmaceutical Industry
Large Behavioral Model (LBM): A Revolutionary Opportunity for the Pharmaceutical Industry
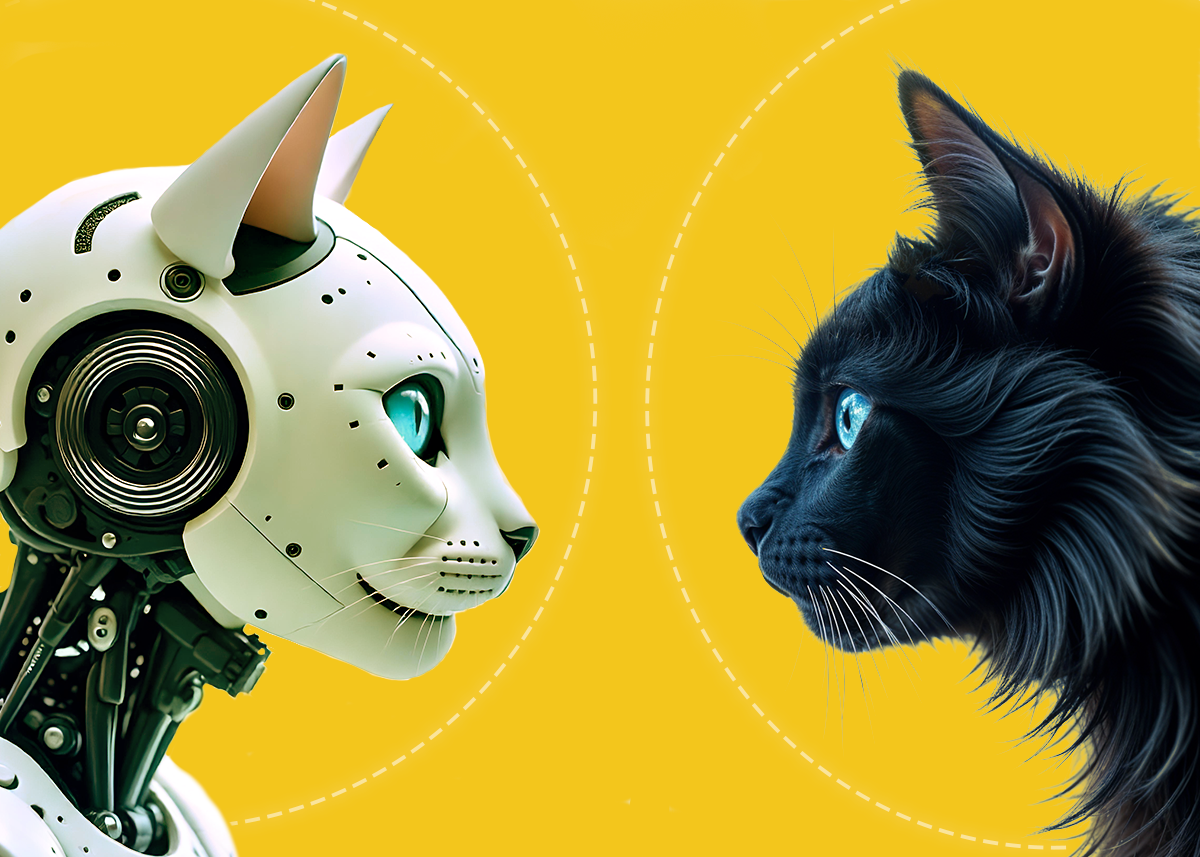
Picture a world where treatments for once incurable diseases are discovered not in years, but in just a few months. What once seemed unachievable is now becoming a concrete reality, thanks to the astounding advances of artificial intelligence (AI). In the pharmaceutical industry, AI has not only accelerated research and drug discovery, but it has also redefined the entire process. But while Large Language Models (LLMs) have grabbed the headlines, another, even more impactful revolution is underway: Large Behavior Models (LBMs). These advanced learning models don’t just speed up processes – they’re transforming the way the industry understands and anticipates patient needs, optimizing manufacturing and reinventing communication with healthcare professionals. The time has come for pharmaceutical groups to seize this unprecedented opportunity, which could well redefine the very foundations of medical innovation!
Types of machine learning and their application in the pharmaceutical industry
Large Language Models (LLM)
Large Language Models (LLMs) are AI models designed to understand and generate natural language. Using massive corpora of textual data, these models perform various tasks such as text generation, machine translation, document classification, or answering complex questions. In the pharmaceutical industry, these models are beneficial for processing massive volumes of data from clinical research, scientific publications, or medical databases. Take the example of AstraZeneca, which, in collaboration with AI companies such as BenevolentAI, has integrated artificial intelligence into its research processes to rapidly identify potential treatments. This collaboration has accelerated the discovery of new drugs for diseases such as idiopathic pulmonary fibrosis, optimizing the research process through massive scientific data analysis.
Although these models are particularly useful for processing textual data, they do not model complex biological interactions or relationships between different behavioral variables, thus limiting their application in in-depth clinical trials or patient management.
Large Behavioral Models (LBM)
Large Behavioral Models (LBMs) represent a major advance, far beyond the capabilities of traditional language models. Unlike purely text-based models, LBMs are capable of simulating complex human behaviors, incorporating learning algorithms to understand how people react in various situations, and considering physiological, psychological, and environmental factors. Their potential for the pharmaceutical industry is revolutionary. Imagine being able to predict, even before the start of a clinical trial, how a group of patients will react to a new drug. This is exactly what LBMs can do. For example, by analyzing patient behavior and physiological responses, an LBM could simulate the efficacy of a treatment in specific populations. This would not only reduce the risk of costly failures in clinical trials, but also identify potential side effects earlier, saving considerable time and resources.
LBMs are still in the development and experimentation phase. They require massive data not only on health but also on human behavior on a large scale, which makes their implementation in the pharmaceutical sector more complex than that of LLMs.
Relational Learning Models (RLM)
Large Relational Models (LRMs) are characterized by their ability to analyze and model complex relationships between different variables, such as molecules, biological targets or clinical outcomes. Unlike LBM or LLM models, LRMs are specifically designed to explore interactions within large databases, be they molecular networks or genetic relationships. Their potential is particularly powerful in the fields of pharmacological research and personalized medicine.
A report published by MDPI (Multidisciplinary Digital Publishing Institute) highlights that advances in big data technologies and machine learning are having a considerable impact on personalized medicine. These approaches effectively enable the analysis of relationships between genetic mutations and disease, accelerating the discovery of new drugs and the creation of targeted therapies. As a result, treatments can be more individualized according to patients’ genetic profiles, transforming the healthcare sector.
Read Full Article: Innovations in Genomics and Big Data Analytics for Personalized Medicine and Health Care: A Review
Although LRMs are highly effective at analyzing complex relational data, they do have certain limitations. Unlike LBMs or LLMs, they do not model human behavior or language comprehension, which confines them to specific scenarios, mainly in the laboratory or in genomic research. So, while powerful for drug discovery or genetic analysis, they are not suited to tasks involving complex behavioral or linguistic interactions.
Impact of AI and LBMs on drug discovery
Accelerating preclinical research
As we all know, discovering new drugs is no easy task. According to a study by the Tufts Center for the Study of Drug Development, it takes between 10 and 15 years, on average, for a candidate molecule to reach the market, at a cost that often exceeds $2.6 billion per drug. It’s long, it’s expensive, and it’s risky. However, artificial intelligence is transforming this landscape. In just a few years, projects like DeepMind ‘s AlphaFold have made incredible advances. AlphaFold, which has revolutionized protein structure prediction – a crucial aspect in drug design – has accurately predicted over 98% of human protein structures, according to a study published in Nature. This has significantly reduced the initial stages of the drug discovery process, by identifying potential therapeutic targets more quickly.
LBM models, on the other hand, take this revolution one step further. Whereas conventional machine learning (ML) models focus on identifying specific therapeutic targets, LBMs have the capacity to process massive quantities of data and model complex human behaviors. In other words, these models not only predict the immediate effects of a treatment but can also anticipate long-term side effects. This drastically reduces the time and cost of drug development, enabling risks to be anticipated even before clinical trials begin.
In fact, according to Pfizer Vice President Enoch Huang, “AI and ML models are turbocharging drug discovery and development. It is already assisting our scientists in proposing new therapeutic hypotheses, predicting patient response to therapy and designing large and small molecule therapies.”
Optimizing clinical trials
Clinical trials account for a huge proportion of expenditure for the pharmaceutical industry, with costs reaching an average of $1.4 billion per trial. It’s a colossal investment, but AI is starting to change the game by optimizing this end-to-end process. Medidata, a company specializing in clinical trial management, is already using predictive AI models to select patients most likely to respond positively to a treatment. This not only reduces the size of trials, but also speeds them up. In 2022, Medidata demonstrated that using AI to fine-tune patient selection can have a significant impact on trial efficiency, reducing both time and costs.
But that’s not all. The introduction of LBM Models may well revolutionize this approach even further. These models are capable of modeling complex human behaviors to create virtual clinical trials before they even begin. This means that we could simulate various scenarios, adjust protocols accordingly, and predict outcomes before committing millions of dollars to a real trial.
“By predicting patient responses and identifying optimal patient populations, these tools enhance trial efficiency and success rates.” — Latshaw, expert in IA in Pharma Industry
AI and pharma manufacturing
Artificial intelligence is gradually establishing itself as a key lever for optimizing the pharmaceutical supply chain, a field where complexity is paramount. Drug manufacturing requires continuous monitoring to ensure product quality and efficacy. Today, AI systems developed by companies such as Siemens and Honeywell already enable real-time analysis of production data. This helps teams spot bottlenecks or detect quality problems before they even occur. These innovations reduce costly downtime, maximizing productivity.
An excellent example of the impact of AI in pharmaceutical production is Pfizer’s use of it in the manufacture of its COVID-19 vaccine. Thanks to AI, Pfizer has been able to reduce production lead times, speeding up the delivery of vaccines worldwide, while ensuring consistent levels of quality.
But the future of optimization could go even further with LBMs. These models could make it possible to simulate complete production lines before products are even manufactured, enabling problems to be anticipated and solved before they occur, reducing waste and increasing efficiency at every stage. For an industry seeking to minimize costs and maximize profitability, LBMs offer an unprecedented opportunity.
Siemens is already experimenting with solutions that use AI to monitor machines and identify potential breakdowns or inefficiencies long before they affect production. This not only maintains a continuous flow, but also saves millions in downtime costs.
Marketing and communication automation in the pharma industry
Artificial intelligence has created a new era in marketing and communications automation for pharmaceutical companies.
- Reduced marketing campaign costs: Analytical tools, such as those offered by Salesforce or Adobe, enable pharmaceutical companies to personalize their campaigns according to the consumption habits and preferences of healthcare professionals. For example, an AI algorithm can analyze thousands of interactions and adjust a campaign in real time to maximize its effectiveness. This significantly reduces costs while improving ROI.
- Automating regulatory communications: Complying with international regulations is a complex requirement for pharmaceutical companies, particularly when they operate in several markets. For example, tools like Veeva Vault are used to monitor regulatory changes in different countries and automatically adjust internal procedures. This ensures that companies remain compliant with local regulations while avoiding costly fines.
But that’s just the beginning. What would you think of a system capable of fully automating marketing and communication campaigns, while tailoring each message to a specific individual, whether consumer or healthcare professional? LBMs make this possible. These models can predict user behavior, adjust messages in real time, and even anticipate market trends or regulatory changes. Campaigns would be more precise, better targeted and even less costly. For example, by anticipating future regulatory changes, a pharmaceutical company could adjust its procedures even before an official change takes place, thus reducing the risk of non-compliance.
Yann LeCun’s artificial intelligence: A model of general cognition
Yann LeCun, Scientific Director of AI at Meta, is working on the development of a model for self-supervised artificial intelligence. Unlike current LLM approaches, which require huge quantities of labeled data, LeCun proposes an approach inspired by the human brain, capable of learning autonomously from unstructured data. The model he proposes seeks to go beyond specialized tasks (such as language processing or behavioral simulation) to create a general-purpose system that could mimic the flexibility of human cognition.
But how could this revolutionize drug discovery? LeCun’s proposed model could simultaneously process genomic, clinical, behavioral and textual data. For example, in a clinical trial, a self-supervised AI could analyze patients’ health data, lifestyle habits and environment to offer more personalized and effective treatments. Imagine an AI capable of suggesting adjustments to a treatment based on a patient’s social behavior or eating habits – information often overlooked in current models.
This general intelligence is not designed specifically for LBMs, but its unsupervised learning capabilities would enable it to model social and behavioral aspects of the patient. This could transform the way drugs are developed and tailored to individual needs. Using large sets of unstructured data, such as electronic health records or doctors’ notes, this AI could identify patterns or correlations invisible to the human eye that could improve patient care.
Yann LeCun‘s artificial intelligence, with its self-supervised, human brain-inspired approach, has the potential to transform the pharmaceutical industry by combining flexibility and generalist learning capabilities with multi-dimensional data analysis. Although it is not specifically designed for behavioral simulations (LBM) or complex relationships (LRM), its flexibility and global approach make it an extremely promising tool for pharmaceutical groups and the healthcare sector.
Large Behavior Models (LBMs) represent not just a technological breakthrough, but a real turning point for the entire pharmaceutical sector. Thanks to their ability to model complex human behaviors and analyze multi-dimensional data, they bring unprecedented flexibility to the research and discovery of new treatments. By integrating data from a variety of sources (genomic, clinical, behavioral), LBMs can anticipate side effects, optimize clinical trials, and personalize patient care to an unprecedented degree.
The potential impact of these technologies is immense: faster, safer clinical trials, more efficient pharmaceutical production chains, and reduced drug development costs. For pharmaceutical groups, LBMs represent an unprecedented opportunity to rethink the way they work and innovate. However, their widespread adoption will require overcoming challenges, particularly in terms of access to behavioral data and systems integration.
Companies that grasp this revolution will undoubtedly be at the forefront of innovation in the years to come, redefining not only modern medicine, but also the way care is designed and delivered on a global scale. The future of the pharmaceutical industry is undeniably shaped by these advanced AI models, and there is no doubt that their influence will be felt ever more strongly as technologies continue to evolve.